Artificial Intelligence (AI) in Machine Manufacturing: Revolution at Every Level
Discover how Artificial Intelligence is transforming machine manufacturing, driving efficiency, revenue growth, and precision. From predictive maintenance to AI-powered pricing, explore real-world applications and learn how to successfully integrate AI into your operations with our essential checklist.
Artificial Intelligence (AI) in Machine Manufacturing: Revolution at Every Level
Artificial Intelligence (AI) is playing an increasingly central role in machine manufacturing, revolutionizing traditional processes and unlocking new potential for revenue and efficiency. AI enables companies in the manufacturing industry to make more accurate predictions, optimize production workflows, and improve the quality of their products.
However, implementing AI requires careful planning and integration to be successful. Many examples today demonstrate how AI-powered business intelligence can be effectively applied in machine manufacturing. This ranges from optimizing manufacturing processes to predictive maintenance and AI-driven pricing of parts portfolios. The implementation of AI requires careful planning and integration to be successful. In our checklist, you'll learn what to consider when introducing AI in machine manufacturing.
What does AI Mean for Modern Machine Manufacturing?
At its core, Artificial Intelligence (AI) represents the ability of machines to take on tasks that traditionally require human thinking and decision-making. AI manufacturing solutions have evolved from a futuristic concept into a practical reality, transforming the entire production process.
Traditional automation solutions, used in industrial manufacturing for decades, rely on pre-programmed instructions. Machines execute predefined tasks with high precision but reach their limits when unforeseen problems or variations occur. This is where AI comes in. Through the use of machine learning and deep learning algorithms, modern systems can learn from past experiences, identify patterns, and make decisions independently based on these insights.
Industrial robots, which were traditionally programmed for repetitive tasks, are now capable of handling complex, non-linear tasks. Thanks to AI, they can autonomously identify, grasp, and integrate various objects into production processes without needing every scenario to be explicitly programmed in advance. These AI manufacturing solutions bring unprecedented levels of flexibility and adaptability to modern manufacturing.
Machine Learning vs. Deep Learning
AI in machine manufacturing incorporates technologies such as machine learning and deep learning, which fuel AI-powered business intelligence. These technologies lay the groundwork for recognizing patterns in large datasets and making informed predictions to improve operations.
Machine Learning
… is a subset of AI that focuses on developing algorithms that can improve themselves using experience and data. In manufacturing, machine learning is used to optimize production processes by identifying patterns in production data and using them to enhance workflow efficiency. For example, machines can learn to automatically adjust their own parameters to enhance production efficiency and reduce energy usage.
Deep Learning
… goes one step further by using complex neural networks capable of recognizing deeper patterns in large datasets. This enables systems to generate precise predictions or make decisions that would have previously required human intervention. A typical example of deep learning in manufacturing is predicting machine breakdowns using sensor and production data.
The Benefits and Current Use of AI in Machine Manufacturing
The adoption of AI solutions in manufacturing represents a fundamental shift, extending far beyond traditional automation. AI has the power to enhance nearly all areas of production by delivering deeper insights, quicker response times, and unparalleled efficiency. In modern manufacturing industry, AI plays a pivotal role, transforming the way companies manage their production operations.
The Importance of AI in Machine Manufacturing Cannot Be Underestimated
AI solutions range from optimizing production lines to fully automating complex manufacturing processes. A key feature of AI is its capacity to analyze enormous data sets and extract meaningful insights. This data, sourced from sensors, machinery, and production systems, is processed in real time, enabling immediate adjustments and improvements.
AI’s significance cannot be emphasized enough. By utilizing machine learning to streamline processes and making decisions based on data analysis, businesses can start to lower production costs, enhance quality, and optimize supply chain efficiency. In a competitive market where speed and accuracy are key to success, AI offers a significant competitive edge. This applies across various fields, such as management, maintenance, and pricing.
20% Increase in Supply Chain Efficiency
By optimizing processes, automating tasks, and improving forecasting accuracy, companies can boost the efficiency of their supply chains by up to 20%, leading to substantial cost reductions and increased market competitiveness.
AI Tools Enable Predictive Maintenance
Predictive maintenance is one of the most promising AI applications in machine manufacturing and a key revenue driver in the after-sales sector. Traditionally, machines are maintained at fixed intervals, often leading to unnecessary downtime or, in the worst-case scenario, unforeseen failures. AI allows maintenance to be carried out precisely when it is required.
By using IoT sensors that continuously collect data on machine conditions and AI algorithms that analyze this data in near real-time, potential failures can be detected early. This allows for predictive maintenance planning, increasing machine availability and reducing maintenance costs. Companies like Siemens and GE have already successfully implemented predictive maintenance to maximize the reliability of their machines while minimizing operational costs.
AI Supported Market-Based Pricing for the Entire Parts Range
AI offers a transformative approach to pricing in the machine manufacturing industry. By leveraging AI to analyze market trends, production expenses, and other key data, companies can adjust pricing for their entire parts inventory according to market data. This enables businesses to respond more quickly to market changes while maximizing profitability.
For instance, MP ONE, the product solution from MARKT-PILOT, offers AI-supported market- and value-based pricing intelligence to optimize the entire parts portfolio. It enables the identification of growth opportunities and the rapid and precise implementation of price changes across thousands of parts, even when market data is insufficient. This shows how AI in machine manufacturing not only improves processes but also specifically leads to increased revenue in the parts business.
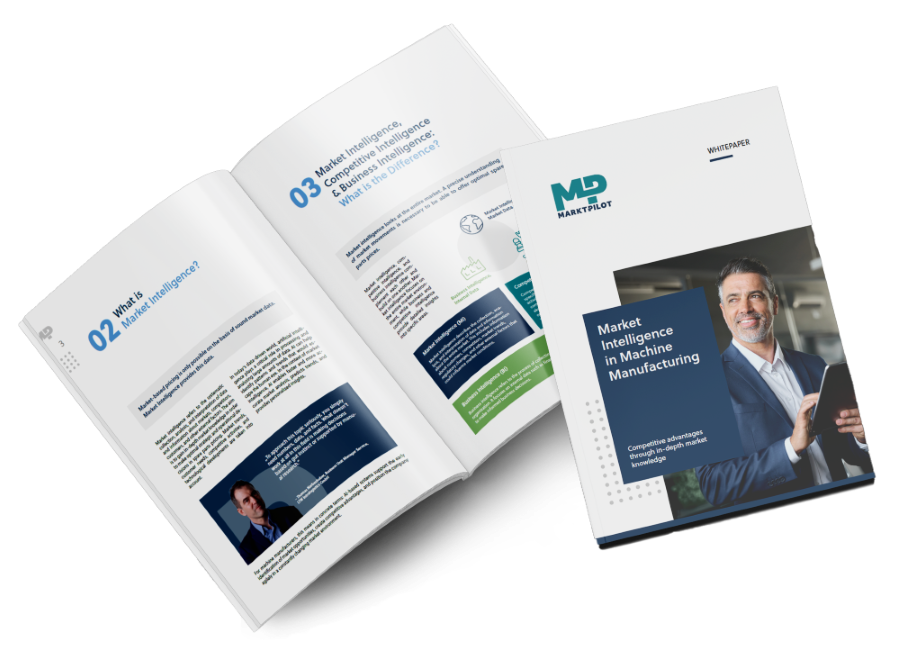
Market Intelligence and AI in Machine Manufacturing
In our whitepaper Market Intelligence in Machine Manufacturing, you will learn everything about market intelligence and the role AI plays in using market data in machine manufacturing, particularly in the aftermarket and parts pricing.
How Artificial Intelligence is Changing Machine Manufacturing
Artificial Intelligence in machine manufacturing is no longer a vision of the future, even though it often still appears that way. In fact, AI solutions already play a crucial role today in optimizing processes at various levels. Many companies worldwide have successfully integrated AI into their operations, particularly in manufacturing, setting new benchmarks for innovation and efficiency.
In machine manufacturing, this means that AI systems can collect data from various sources, analyze it, and make independent decisions based on it. The use of these solutions not only offers potential for process optimization and automation but also ensures long-term competitiveness in the competitive mechanical engineering industry.
These five companies are considered global pioneers in the development and application of AI solutions.
Use Cases – Successful Implementation of AI Solutions in Machine Manufacturing
- Siemens: The German company Siemens uses AI to revolutionize its production processes. Through its MindSphere platform, Siemens has developed a system that analyzes production data in real time and identifies optimization opportunities. This enables flexible adjustments to production lines, dramatically boosting efficiency in response to shifting demands.
- Caterpillar: Caterpillar uses AI algorithms to predict the demand for spare parts in different regions and for various types of machines. By analyzing operational data, machine usage, and historical orders, Caterpillar optimizes inventory in their warehouses, enabling faster response to customer needs. This reduces storage costs while ensuring high availability of spare parts.
- John Deere: John Deere integrates AI-based technologies into its "John Deere Operations Center" platform. This platform allows farmers and machine operators to monitor their equipment in real-time and receive maintenance forecasts. AI-driven analyses also predict the need for spare parts in advance, enabling John Deere to respond quickly and efficiently to maintenance requirements.
- General Electric: GE uses AI for its "Predix" platform, which is used in the aviation, energy, and manufacturing industries. The platform collects and analyzes data from machines to determine the best times for maintenance. Based on this data, maintenance can be optimized and spare parts can be ordered in a timely manner. This minimizes downtime and optimizes maintenance costs.
- Kärcher Municipal: Kärcher has implemented a market-oriented pricing strategy for its spare parts business using AI. With the solution from MARKT-PILOT, Kärcher Municipal gains a clear and transparent market overview of current purchase part prices. This makes it immediately apparent which parts are over- or undervalued. The automated market price research serves as the basis for market-oriented spare parts pricing and enables intelligent price adjustments
Implementing AI at Machine Manufacturers
The effectiveness of artificial intelligence in the machine manufacturing sector is closely tied to the quality of the data it uses. AI systems need vast amounts of data to learn and generate precise predictions. If the data is not high quality, there is a potential risk that the AI may deliver incorrect or inconsistent results.
Data Quality, Availability, and Accessibility
For successful AI implementation in machine manufacturing, identifying relevant data sources is essential. This includes sensor data, production histories, and supply chain information. This data must not only be available in sufficient quantity but also of high quality. Preparing and cleansing the data is a vital step to ensure that AI models work with reliable information.
In addition to data quality, the continuous availability and accessibility of data are also crucial. By leveraging IoT technologies and edge computing, companies can ensure that relevant data is captured and processed in real time. One challenge is data silos. These can be avoided by integrating and harmonizing data from various departments and systems. This creates a comprehensive overview of production processes, ultimately improving the decision-making process with the help of AI.
Choosing the Right AI Solution for Machine Manufacturing
Selecting the appropriate AI systems is a crucial step toward the successful implementation of AI in machine manufacturing. It’s important to find a solution that not only addresses the company’s present needs but is also able to scale and be ready for future developments.
Assessing Needs
First, it’s essential to identify the specific needs of the company. What problems should be solved through the use of AI? In which areas of production or management is there potential for optimization? These questions are key to defining the requirements for an AI solution. At the same time, an evaluation of internal capacities should be conducted to determine if the company has the necessary expertise and infrastructure to successfully implement AI.
Criteria for Selecting AI Solutions
The solution must be able to seamlessly integrate with existing systems and processes. It is important that it is compatible with the existing IT infrastructures and does not require extensive changes or adjustments.
The solution must be adaptable to changing production conditions and business requirements. It should be capable of handling larger data sets and meeting higher performance requirements as they grow.
The solution should be easy to use and intuitively understandable. A complex user interface or extensive training can lead to lower acceptance and usage of the solution.
Since AI solutions often process sensitive data, it is essential to implement strict data protection and security measures. There should be clear guidelines for handling data and mechanisms to ensure data security.
An AI solution is only as good as its support team. It's important to ensure that the provider has a competent and reliable support team available quickly for any issues or questions.
The costs of the solution must be carefully evaluated. It's important to consider the total costs over the entire lifecycle, including implementation, maintenance, and potential upgrades. While a high-quality AI solution may initially be more expensive, it often provides higher profitability in the long run.
These criteria help ensure that the chosen AI solution not only meets current needs but also provides long-term value. It is important to consider the solution not in isolation, but in connection with existing IT systems and the future business strategy. Only in this way can it be ensured that the AI solution is a meaningful addition to the overall strategy of the company.
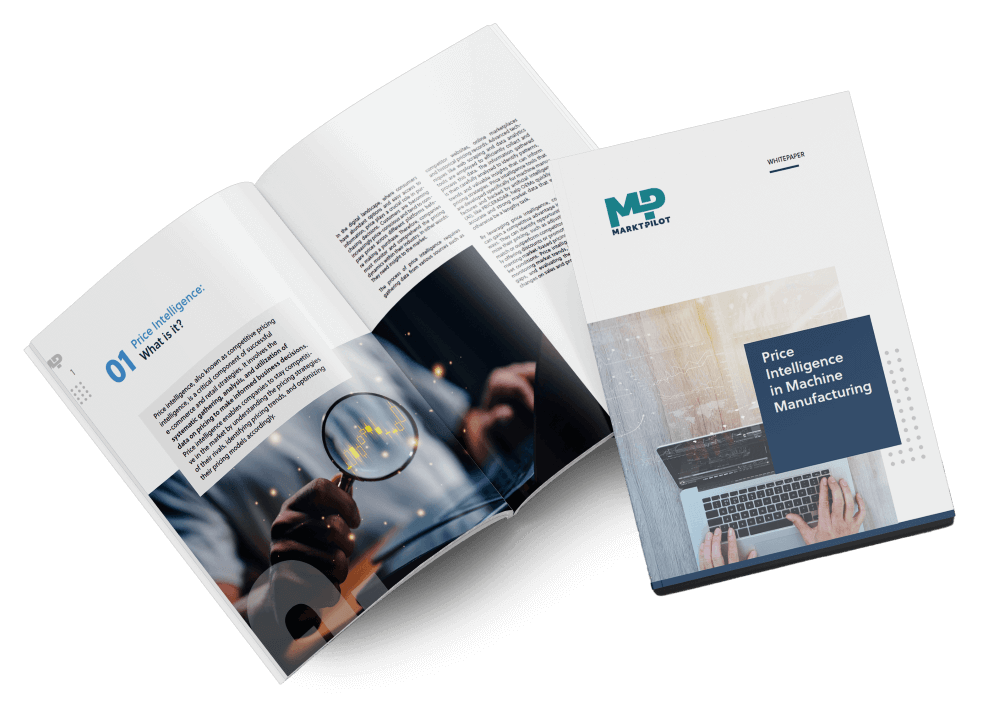
With AI to More Price Intelligence in Machine Manufacturing
In our whitepaper Price Intelligence in Machine Manufacturing, you'll gain necessary knowledge and insights related to price intelligence. Learn how to use AI solutions to make pricing in the parts business even more effective and profitable.
Assessing ROI and Performance Metrics of AI Solutions
Once an AI solution is in place, it is essential to evaluate the success and return on investment (ROI). This requires identifying and regularly tracking relevant key performance indicators (KPIs).
Identifying Critical KPIs
Key KPIs for AI implementation typically include productivity metrics that capture efficiency improvements enabled by AI, as well as quality assurance metrics that show how AI enhances product quality and reduces defects. Maintenance efficiency can also be assessed by evaluating the savings and improvements achieved through predictive maintenance.
When evaluating the ROI of an AI implementation, it is essential to perform a thorough cost-benefit analysis. This process compares the costs of implementation with the savings and productivity improvements generated by AI. In addition to short-term effects, long-term impacts of AI implementation should also be considered to get a complete picture of profitability.
- OEE (Overall Equipment Effectiveness): A key KPI that measures the overall efficiency of equipment. OEE is composed of three factors: availability, performance, and quality. By using AI to optimize production processes, OEE can be significantly improved, leading to higher machine utilization and efficiency.
- Cycle Time: A key KPI that measures the overall efficiency of equipment. OEE is composed of three factors: availability, performance, and quality. By using AI to optimize production processes, OEE can be significantly improved, leading to higher machine utilization and efficiency.
- Machine Utilization: Utilization reflects how intensively machines are used in relation to their maximum capacity. AI-driven optimizations can enhance utilization by improving efficiency and minimizing downtime.
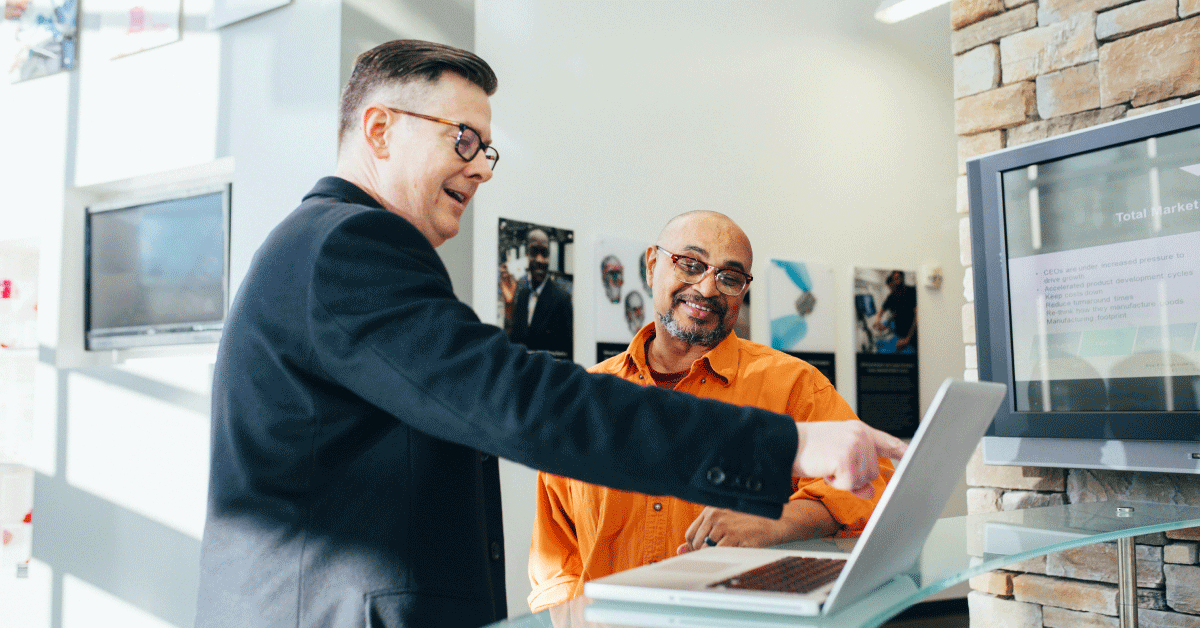
- Defect Rate: This KPI tracks the proportion of manufactured parts that fail to meet quality requirements and must be rejected. AI can assist in lowering the defect rate by identifying and addressing quality deviations at an early stage.
- First Pass Yield (FPY): FPY measures the percentage of products that meet quality standards on the first pass, without requiring rework. A high FPY indicates efficient and error-free production. AI can contribute to improving FPY by continuously analyzing data and optimizing production parameters.
- MTBF (Mean Time Between Failures): This KPI measures the average operational time between two machine failures. AI-powered predictive maintenance can boost MTBF by forecasting breakdowns and suggesting preventative maintenance measures.
- MTTR (Mean Time to Repair): MTTR indicates the average time required to repair a failed machine and get it back into operation. AI can help reduce MTTR by delivering precise diagnostic data and streamlining the repair process.
- Cost per Unit: This KPI measures the production cost for each unit produced. AI can reduce these costs by optimizing processes and decreasing material and energy consumption.
- Maintenance Expenses: Utilizing AI for predictive maintenance can greatly decrease unexpected maintenance costs, as potential failures are identified and resolved in advance.
- Reduced Downtime: Predictive maintenance minimizes machine downtime, increasing productivity while also reducing operational costs due to less idle time.
- Price Optimization: By using MARKT-PILOT’s pricing software, companies can automate their price research and adjustments, leading to a more efficient pricing strategy, higher margins, and increased revenue. At the same time, manual processes are reduced, resulting in a sustainable improvement in operational efficiency.
- On-Time Delivery (OTD): This KPI measures the percentage of orders delivered on time. AI can optimize the supply chain by improving forecasts for material procurement and aligning production with demand.
- Inventory Management: AI can assist in optimizing inventory by enabling more accurate demand planning, helping to prevent both excess inventory and stock shortages.
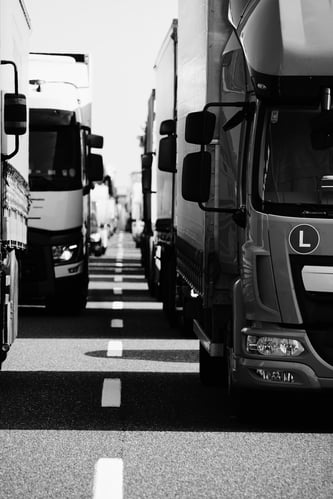
Customer Success Story: LTW Intralogistics GmbH
THE CHALLENGE
Static price lists and reactive price adjustments no longer meet current market demands, which is why the traditional cost-plus logic needs to be rethought. Especially in dynamic and volatile times, one of the greatest challenges in the service sector is meeting the continuously changing customer requirements.
Thomas Narbeshuber, Business Unit Manager Service at LTW Intralogistics GmbH, aptly summarizes the current situation in service: "The challenge in after sales is to meet the constantly changing requirements of customers as a logistics supplier, especially in increasingly dynamic and volatile times." But how can these processes be made more efficient and profitable?
INNOVATIVE PRICING STRATEGIES TO FOSTER TRUST
The solution? To maintain competitiveness, an intelligent pricing strategy is imperative. This entails creating a simple pricing model seamlessly integrated into existing processes and easily maintained.
With the implementation of MARKT-PILOT, LTW Intralogistics has adopted a lean and scalable solution, positioning the company as a fair and reliable partner for its customers in terms of both availability and prices.The software's swift deployment without the need for extensive implementation enables a quick start.
Outlook: AI in Machine Manufacturing
The integration of AI-powered business intelligence into machine manufacturing opens up numerous possibilities for process optimization, automation, and efficiency improvements. AI helps businesses automate complex workflows and streamline their manufacturing operations, leading to a significant competitive advantage.
In addition, AI enables precise, data-driven pricing strategies, making it easier for companies to adapt to dynamic market changes and increase their profitability. By turning data into actionable insights, AI promotes strategic growth and supports informed decision-making. These benefits make businesses more efficient, agile, and competitive, which is particularly valuable in today’s increasingly demanding market environment.
Investing in AI technologies is therefore not only a step toward modernization but also a strategic advantage that positions companies for long-term success.
FAQs
What Is Artificial Intelligence (AI) in Machine Manufacturing?
Artificial Intelligence in machine manufacturing involves the use of algorithms and machine learning to automate processes, analyze data, and make informed decisions. Examples include predictive maintenance, quality control, and process optimization.
Why Is AI Important in Machine Manufacturing?
AI increases efficiency, reduces downtime, and optimizes use of resources. Machine manufacturers can gain a competitive advantage, lower production costs, and enhance their innovation capabilities with AI.
How Does AI Work in Machine Manufacturing?
AI uses data from sensors, IoT devices, and production systems to identify patterns and make data-driven decisions. For example, machines can autonomously detect errors or suggest process enhancements. Maintenance and service around the machines can be improved.
What Are the Benefits of AI in Machine Manufacturing?
The key advantages of AI in machine manufacturing include:
- Predictive Maintenance: Predicting maintenance needs before failures occur.
- Automation: Reducing manual processes.
- Quality Control: Automatically detecting production defects.
- Efficiency Gains: Improved resource usage, higher revenues and shorter production times.
Where Is AI Used in Machine Manufacturing?
In machine manufacturing, AI is typically used successfully in the following areas:
- Production: Optimizing production lines.
- After-Sales Service: Automating spare parts management and pricing stragies and enabling predictive maintenance.
- Logistics: Optimizing routes and inventory management.
- Product Development: Enhancing simulations and prototype designs.
Who Benefits from AI in Machine Manufacturing?
Machine manufacturers, suppliers, and customers all gain advantages. Companies enjoy increased efficiency and cost savings, while customers benefit from more reliable products and improved service.
When Does It Make Sense to Use AI in Machine Manufacturing?
AI in machine manufacturing makes sense when companies:
- Generate large amounts of data that need analysis.
- Want to automate repetitive manual tasks.
- Aim to stay competitive by adopting cutting-edge technologies.